Understanding Contextual Decision-Making in AI through Vector and Graph Models
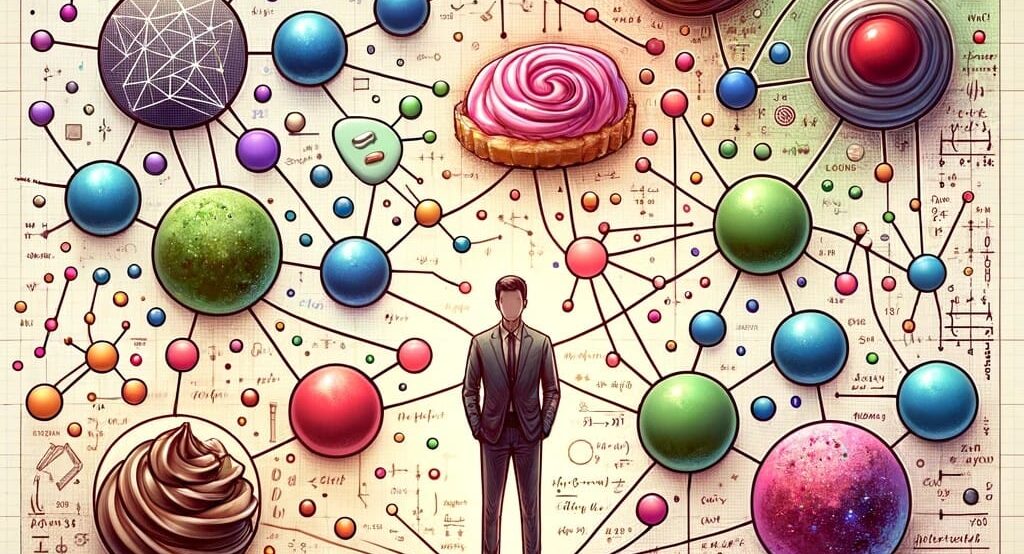
In the realm of artificial intelligence (AI), understanding human behavior and decision-making processes has always been a fascinating challenge. A simple yet profound scenario—a person with a sweet tooth craving a specific sweet from a particular shop, only to find it unavailable—offers a perfect illustration of the complexity AI models face in mimicking human decision-making. This individual’s choices, which may vary from: – avoiding the sweet altogether, – selecting an alternative sweet from the same shop, or – seeking the desired sweet at another shop highlight the nuanced and context-dependent nature of human decisions. So , let’s dive in the very challenge posed while making contextual Decision-Making The key to replicating this decision-making process in AI lies in the ability to model not just the decisions themselves, but the context and factors influencing these decisions. Contextual decision-making in AI is about understanding the ‘why’ behind each choice and the factors that alter this decision in different directions. It requires a model that can weigh various factors, such as urgency, preference, availability, and past experiences, to predict or decide in a manner that aligns with human behavior. Welcome! Vector Models – best suited for understanding preferences and similarities. Vector models, particularly those based on embedding techniques like Word2Vec or GloVe, offer a way to represent entities (such as sweets, shops, or preferences) in a multi-dimensional space. In this space, the distance and direction between vectors can signify similarity or preference. For example, different sweets can be represented as vectors in this space, and a person’s preference can be modeled as a vector pointing towards their favored sweet. The absence of the desired sweet at the preferred shop can be represented as a vectorial deviation, and the AI’s task is to find the vector (decision) that realigns with the person’s preference vector as closely as possible under the given context. Vector Models fight for their superiority against Graph Models used for mapping relationships and influencing factors. Graph models excel at representing complex relationships and dependencies between entities and decisions. In our scenario, a directed graph could model entities (people, sweets, shops) as nodes and the relationships or decisions (preferences, availability, choices) as edges, with weights representing the strength or importance of these relationships. Factors like urgency and importance can dynamically adjust these weights, influencing the decision path the model predicts or recommends. For instance, a graph model can represent the decision-making process as a pathfinding problem. Nodes represent the person, potential sweets, and shops, while edges represent the possible decisions (e.g., choosing a different sweet, going to another shop). The weights on the edges could be influenced by factors such as urgency (how badly does the person want the sweet?) or convenience (how easy is it to go to another shop?), thus guiding the AI in predicting the most likely decision under the given circumstances. Let’s add the spice called Contextual Awareness to this recipe for making it AI driven. To effectively mimic human decision-making, AI models need to integrate context awareness, understanding that the same person might make different decisions under different circumstances. This involves dynamically adjusting the model’s parameters (vector directions, graph edge weights) based on the current context, past behaviors, and the relative importance of various factors including personas. I’ll try to conclude this interesting topic by just highlighting the underlying mathematical foundations which exist for decades if not centuries. The mathematical models underpinning these AI decision-making processes involve complex algorithms for vector space manipulation and graph theory. Techniques such as cosine similarity for vector models or Dijkstra’s algorithms for graph models can be used to evaluate decision options and predict outcomes. Of course fine-tuning these is nothing less than a perfect blend of art and science where human and machine intelligence marry each other to produce a wonderful product. So , in conclusion , the sweet shop dilemma underscores the importance of context and variability in human decision-making. By leveraging vector and graph models, AI can better understand and predict human behavior, offering insights not just for simple decisions but for complex, context-driven choices that define our everyday lives. As AI continues to evolve, the ability to accurately model and respond to the nuanced preferences of individuals will be crucial for developing more intuitive, human-like AI systems and one day in the distant future the walls will diminish. Happy Reading!! Author – Sumit Rajwade, Co-founder: mPrompto
Friends like Horses and Helicopters
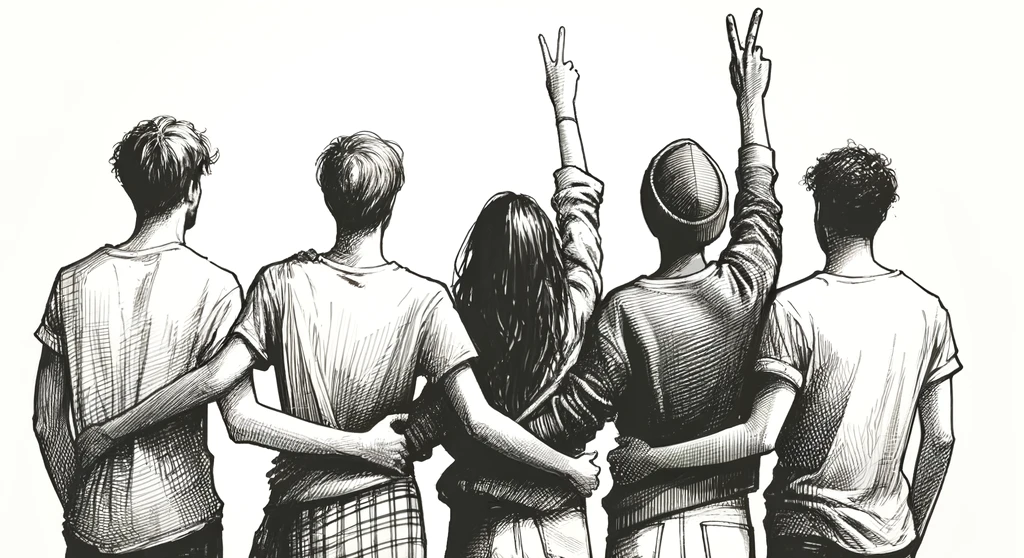
The other day, I was watching my daughter learn about word associations by my wife, Aditi. Aditi was explaining the concept of “friends of letters,” where each letter is associated with words that start with it, much like “H” has friends in “horse” and “helicopter.” This got me thinking about the world of generative AI, a field I find myself deeply immersed in. Just like how my daughter is taught to associate letters with words, generative AI operates on a similar principle, predicting the next word or phrase in a sequence based on associations and patterns it has learned from vast datasets. Now, the analogy of letter friends is a great starting point to help someone grasp the basics of how generative AI works. However, it’s a bit simplistic when we consider the full complexity of these models. Generative AI doesn’t just consider the “friends” or associations of a word. It also takes into account the context of the entire sentence, paragraph, or even document to generate text that is coherent and contextually relevant. It’s like taking the concept of word association to a whole new level. So, when the AI sees the word “beach,” it doesn’t just think of “sand,” “waves,” and “sun” as its friends. It also considers the entire context of the sentence or paragraph to understand the specific relationship between “beach” and its friends in that situation. Let’s take a moment to delve deeper into how a Large Language Model (LLM) like GPT-3 works. When you input a phrase or sentence, the model processes each word and its relation to the others in the sequence. It analyzes the patterns and associations it has learned from the vast amounts of text in its training data. Then, using complex algorithms, it predicts what the next word or phrase should be. It’s a bit like a super-advanced version of predictive text on your phone, but on steroids. But here’s where it gets tricky. These models are, at the end of the day, making educated guesses, and sometimes, those guesses can be off the mark. We’ve all had our share of amusing auto-correct fails, haven’t we? Let me try to explain it with an example in a B2B scenario, particularly in drafting emails or reports, which is a common task in the business world. Imagine you’re a sales professional at a B2B company and you’re tasked with writing a tailored business proposal to a potential client. You start by typing the opening line of the proposal into a text editor that’s integrated with an LLM like GPT-3. As you begin typing “Our innovative solutions are designed to…” the LLM predicts and suggests completing the sentence with “…meet your company’s specific needs and enhance operational efficiency.” The model has learned from a myriad of business documents that such phrases are commonly associated with the words “innovative solutions” in a sales context. However, the trickiness comes into play when the LLM might not have enough industry-specific knowledge about the potential client’s niche market or the technical specifics of your product. If you just accept the LLM’s suggestions without customization, the result might be a generic statement that doesn’t resonate with the client’s unique challenges or objectives. For instance, if the client is in the renewable energy sector and your product is a software that optimizes energy storage, the LLM might not automatically include the relevant jargon or address industry-specific pain points unless it has been fine-tuned on such data. So, while the LLM can give you a head start, you need to guide it by adding specifics: “Our innovative solutions are designed to optimize energy storage and distribution, allowing your renewable energy company to maximize ROI and reduce waste.” Here, the bolded text reflects the necessary B2B customization that an educated guess from an LLM might not get right on its own. This illustrates the importance of human oversight in using LLMs for B2B applications. While these models can enhance productivity and efficiency, they still require human expertise to ensure that the output meets the high accuracy and relevancy standards expected in the business-to-business arena. My next blog will cover just that and help you understand how a ‘Responsible Human in the Loop’ makes all the difference for an organization while working with some use cases of generative AI. I’m really looking forward to diving deeper into the realm of human dependency in using Generative AI my subsequent blog post, where we’ll explore how they add a layer of safety and relevance to the responses generated by LLMs. So, stay tuned! If you think you have understood Generative AI better with this blog, do drop me a line. I always look forward to a healthy conversation around Generative AI. Remember, the world of AI is constantly evolving, and there’s always something new and exciting just around the corner. Let’s explore it together! Author – Ketan Kasabe, Co-founder: mPrompto